Day 2 :
Session Introduction
Stan Christiaens
Vrije Universiteit of Brussels,Belgium
Title: Unearthing Valuable Data Insight via an Amazon-ified Approach to Data Discovery and Data Analytics
Biography:
Stan leads the Collibra global product organization with a focus on driving data governance technology innovation. Prior to co-founding Collibra, Stan was a senior researcher at the Vrije Universiteit of Brussels, a leading semantic research center in Europe, where he focused on application-oriented research in semantics. Stan is a sought-after expert resource, industry speaker, and author on the topic of data governance and semantics. He has participated actively in several international research projects (such as ITEA, FP6 and FP7) and conferences (including OTM, FIS and ESTC). He has also published various articles and patents in the field of ontology engineering. Stan holds a Master of Science degree in Information Technology and a Master’s degree in Artificial Intelligence from Katholieke Universiteit Leuven and a Postgraduate in Industrial Corporate Governance from Europese Hogeschool Brussel.
Abstract:
For data to be valuable it needs to be discoverable. Many organizations suffer from an “analytics gap,” a common ailment that occurs when your big data and analytics vision doesn’t live up to its promise. Data scientists and business analysts struggle to find the information they need when they need it, and as seen in a recent survey, they can spend up to 60% of their time cleaning and organizing the data -- preventing them from delivering the important insight and innovation needed for business success. As even more data is brought into the environment, from social media, the Internet of things (IoT) and other sources, requesting access to data sets becomes a herculean task.
Increasingly, however, savvy businesses are recognizing the value of taking an “Amazon-ified” approach to data through a data governance model combined with catalog capabilities. Just as consumer-oriented sites like Amazon and Google offer easy-to-use search capabilities that span multiple information silos, while also providing relevant, curated metadata, this same approach can be applied to data discoverability for users to drive strategic insight, powerful data analytics and smart business decisions.
In this presentation by Stan Christiaens, co-founder and CTO of Collibra, conference attendees will learn:
- How an Amazon-ified approach to finding and accessing data enables data scientists and analysts to more simply uncover valuable data for analytics from one central location, instead of losing time searching for dirty data.
- Having quick and easy access to accurate data through an automated data catalog enables businesses to leverage information, in addition to tapping into the power of the crowd to see which data has proven most useful to which people, resulting in more simplified and timely reporting.
- How data catalog as part of an integrated data governance program, provides a collaborative framework to ensure data accountability and ownership to deliver high-quality data that is easily and consistently accessible to users.
Sumitha P K
International Technological University, USA
Title: Sentiment analysis of Android and iOS using Twitter text mining

Biography:
I am currently pursuing Masters in Computer Science at International Technological University. I also hold a Masters in Electronic System Design with a Gold Medal. I have published an IEEE paper based on my Masters Industrial project at Sanmina SCI. Later, I joined Huawei where I worked in Embedded framework development and testing. I am currently working as an Analyst at ITG America, an Ed-tech company based in San Jose,CA
Abstract:
Twitter is a popular micro-blogging site where users express their opinions about various topics via tweets. The two major Mobile Operating Systems in the Market are iOS by Apple and Android by Google. About 99.6 percent of smartphones use Android or iOS. Apple’s iOS are currently included only with iPhone, iPod and the iPad, where as Google allows Android on any number of devices. Both Operating Systems have come a long way since their introductions, but deciding which of the two Mobile Operating Systems is the best can be difficult. The aim of my research is to perform a lexicon based sentiment analysis of the two major Mobile Operating Systems – Android and iOS by extracting tweets with hash tags Android and iOS. Sentiment Analysis is the process of computationally determining whether a tweet is positive, negative or neutral. It would be very interesting to compare the sentiments over time of the top mobile Operating Systems and also, the leading smartphones: Samsung Galaxy and iPhone.
This research presents an investigation into the sentiments of Android and iOS and the leading smartphones. A sentiment lexicon is used to extract sentiment scores from twitter feeds.
Patrice Bertrand
Université Paris-Dauphine, PSL Research University, PARIS, FRANCE
Title: Multilevel clustering models and dissimilarities
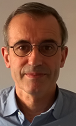
Biography:
Patrice Bertrand received the Ph.D. degree in Applied Mathematics in 1986 from University Paris-IX Dauphine. He is currently an Associate Professor at university Paris-Dauphine. From 1992 to 2013, he was a research collaborator at the French National Institute for computer science and applied mathematics. His research interests focus on ordered sets, clustering structures that extend the classical hierarchical model and allow overlapping clusters, and clustering evaluation. He has authored a number of research papers in international journals and conferences. He was the scientific secretary of the International Federation of Classification Societies (IFCS) from 2011 to 2013.
Abstract:
Overlapping clustering is a clustering structure in which objects may belong to more than one cluster. New multilevel clustering models, which were mostly introduced during the 1980’s, include overlapping clusters and extend he well-known Benzécri-Johnson bijection. This talk is concerned with the characterization of such various multilevel clustering models within the framework of general convexity. Along this line, both the paired hierarchical model and the k-weakly hierarchical models for k ≥ 3, are characterized as interval convexities. Sufficient conditions are provided for an interval convexity to be either hierarchical, paired hierarchical, pyramidal, weakly hierarchical or k-weakly hierarchical. In addition, an algorithm is introduced for computing the interval convexity induced by any given interval operator. A general clustering algorithm is then derived to build any of the previously considered multilevel clustering models. This approach is illustrated by considering specific parameterized interval operators, that can be defined from any dissimilarity index, and selected in an adaptive way.
Laleh Haghverdi
EMBL Heidelberg, Meyerhofstraße , Germany
Title: High-dimensional Single Cell Gene Expression Data and Batch Effect Corrections

Biography:
Laleh Haghverdi has completed a PhD in Mathematics at Technical University of Munich. After a year of postdoctoral fellowship at the European Bioinformatics Institute in Cambridge, she is currently a postdoctoral fellow at the European Molecular Biology Laboratory in Heidelberg.
Abstract:
Emerging about a decade ago, single cell genes expression measurement technologies have facilitated the study of heterogeneous populations of cells such as in development and cell differentiation. Single cell Ribonucleic acid sequencing (scRNA-Seq) techniques can measure the expression level of several thousand genes at the single cell level for millions of cells. Increasingly used by several laboratories, the technique provides a big amount of data which opens new opportunities for knowledge extraction using new machine learning and computational methods. I will discuss the properties of high-dimensional data which needs to be taken care of when dealing with such big expression data, and discuss in an instance on how high-dimension properties allowed us to develop a new method for batch effects correction and data integration across several laboratories.
Andy Handouyahia and Essolaba Aouli
Employment and Social Development Canada , Government of Canada
Title: Increasing the Use of Administrative Databases to Foster Innovation
Biography:
Andy Handouyahia is currently a Manager in the Evaluation Directorate at Employment and Social Development Canada. He is responsible for the methodology and the data. Andy holds a Master of Science degree from the University of Sherbrooke obtained in 1997. Before pursuing his master's degree, he held a degree in Applied Mathematics. He has been teaching for nearly 20 years as a lecturer at the Université du Québec en Outaouais. He worked for over 13 years at Statistics Canada where he was responsible for data collection and processing. Thereafter; in 2009; he joined the Treasury Board Secretariat as Business Solutions.
Essolaba Aouli is currently a Senior Data Analyst with the Evaluation Branch at Employment and Social Development Canada. He is responsible for conducting analyzes and evaluating the potential of the data for the purpose of public policy analysis. Essolaba holds a Master's degree in Economics from the Université Laval obtained in 2012.
Abstract:
The use of administrative data sources for program evaluation has increased in recent years. This paper discusses the improvements in past years in the use of linked administrative data files to facilitate program net impact evaluation. Employment and Social Development Canada (ESDC) has pioneered and implemented the innovative “Medium Term Indicators (MTI)” initiative, consisting of 20-year longitudinal integrated administrative data files from Employment Insurance (EI) and Canada Revenue Agency (CRA) Income Taxes data files. The MTI initiative grants evaluators/researchers the ability to effectively and efficiently measure incremental program impacts over various time periods, using state-of-the-art econometric techniques alongside well-organized, detailed administrative data files. This new initiative is designed to assist decision-making in the context of Government of Canada policy on results, supporting evidence-based evaluation analyses and enabling the examination of many different kinds of labour market research topics. Furthermore, this new approach to labour market program evaluation has also significantly reduced the need to conduct costly surveys and has provided timely evidence-based guidance to support both program and policy development.

Biography:
Jason leads Bonobos' data science team, whose responsibilities include building and maintaining a portfolio of data products to support the business. He spends his time collaborating with people across teams and helping them think about how to use data to solve problems.
Before joining Bonobos he worked as a data scientist in a handful of companies, some early-stage and some later-stage, and as a data science course designer and instructor. His previous work experience was in the financial markets as a derivatives arbitrage trader, and his academic background is in mathematics and theoretical physics.
Abstract:
"Data science" has become nearly a household phrase, but disagreement still remains about the skills a successful practitioner should have.
One area of expertise that is occasionally referred to but consistently underrated is communication; specifically, the ability to exchange technical information for domain-specific information with a non-technical audience.
Taking this responsibility seriously leads to the idea of data products. While some data products rely on sophisticated statistical and engineering techniques, their primary function is to address customer demand. As such, the tools of product development can be directly applied by data scientists to ensure the efficiency of their efforts and the adoption of their solutions.
Prof. Felix T.S. Chan
The Hong Kong Polytechnic University,Hong Kong
Title: Prediction of Flight Departure Delay with Big Data
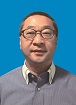
Biography:
Prof. Felix Chan received his BSc Degree in Mechanical Engineering from Brighton Polytechnic, UK, and obtained his MSc and PhD in Manufacturing Engineering from the Imperial College of Science and Technology, University of London, UK. He is the Associate Dean (research) in the Faculty of Engineering, The Hong Kong Polytechnic University. His current research interests are Logistics and Supply Chain Management, Operations Management, Production Management, Distribution Coordination, Systems Modelling and Simulation, Supplier Selection, AI Optimisation, Aviation Manageemnt. To date, Prof. Chan has published over 16 book chapters, over 340 refereed international journal papers and 280 peer reviewed international conference papers.
Abstract:
Flight departure delay is known as a common problem happening every day in every airline and in every airport. However, the impact of flight delay does not only cause huge economy loss to airlines but also indirectly to its dependent industries, including airport and even to the passengers. In the past, flight departure delay estimation is usually studied based on historical data concerning a particular flight or flights in an airport. Numerous of statistical tools or analytical methods have been proposed to increase the prediction accuracy. However, as the data concerned is usually focused and mostly limited to the flight data only. Many indirect factors have not been considered, such as the airport congestion, number of incoming or departure flights, etc. As nowadays, with the mature of many advanced technologies, flight data becomes more accessible and updated. In this connection, the objective of this paper is to propose an Artificial Neural Network (ANN) for flight departure delay prediction by using big data analysis approach. We collected 1 year flight data of Hong Kong International Airport for analysis. We considered various factors as our input variables, including weather, number of arrival and departure flights, holidays, etc. We compared our proposed ANN method with traditional regression based analysis approaches. The results demonstrate that the proposed ANN method outperforms the traditional approaches. This demonstrates the significant impact of considering those indirect factors on the flight departure delay prediction.
Vadim Markovtsev
associate professor at Moscow Institute of Physics and Technology,Russia
Title: Machine Learning on Source Code

Biography:
Vadim is a Google Developer Expert in Machine Learning and a Lead Machine Learning Engineer at source{d} (sourced.tech) where he works with "big code" and natural languages. His academic background is compiler technologies and system programming. He is an open source zealot and an open data knight.
Vadim is one of the creators of the historical distributed deep learning platform Veles (https://velesnet.ml) while working at Samsung. Afterwards, Vadim was responsible for the machine learning efforts to fight email spam at Mail.Ru - the largest email service in Russia. In the past, Vadim was also a visiting associate professor at Moscow Institute of Physics and Technology, teaching about new technologies and conducting ACM-like internal coding competitions.
Abstract:
Machine Learning on Source Code (MLoSC) is an emerging and exciting domain of research which stands at the sweet spot between deep learning, natural language processing, social science and programming. We've accumulated petabytes of source code data which is open, yet there have been few attempts to fully leverage the knowledge that is sealed inside. This talk gives an introduction into the current trends in MLoSC, presents the tools and some of the applications, such as deep code suggestions and structural embeddings for fuzzy deduplication. There is an additional emphasis on mining the “big code”.
Session Introduction
Jose Manuel Lopez-Guede
University of the Basque Country (UPV/EHU),Spain
Title: Data Mining applied to Renewable Energies Industry
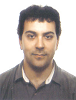
Biography:
Dr. Jose Manuel Lopez-Guede received the Ph.D. degree in Computer Sciences from Basque Country University. He got 3 investigation grants and worked in a company 4 years. Since 2004 he worked as full time Lecturer and since 2012 as Assoc. Prof. He has been involved in 24 competitive projects and published more than 100 papers, 25 on Educational Innovation and the remaining in specific research areas. He has 25 ISI JCR publications, more than 15 other journals and more than 40 conferences. He has belonged to more than 10 organizing committees of international conferences and to more than 15 scientific committees.
Abstract:
One of the key activities of Data Mining is to discover and make clear hidden relations and working rules in complex systems. Renewable energies industry is a complex scope in which first principles approaches have been used to make predictions about the behavior of the different elements which are composed of, but that approach is not enough to deal with complex problems, where more intelligence-based approaches are needed. For example, in the case of photovoltaic energy, Data Mining can be used for autonomous learning of the behavior of elements at different scale of complexity, e.g., photovoltaic cells, photovoltaic panels or modules, photovoltaic arrays or large photovoltaic facilities, in such a way that the obtained models could be used for different purposes. Two of the most usual purposes found in the literature are the prediction of electric energy generation depending on the climatologic conditions, and the possibility of detecting whether the devices in a general sense are at a point of operation that is going far away from the supposed for a given conditions, i.e., the devices are starting to work not properly, being very convenient to take actions from both technical and economical points of view.
Ryan Mandell
Mitchell International, San Diego,California
Title: Bringing Data to the Masses: Strategies to Make Analytics Accessible to Non-Experts
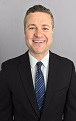
Biography:
Ryan Mandell is the Director of Performance Consulting for Mitchell International, a leading innovative software company that provides solutions to the property and casualty and collision repair industries. In his current role, Ryan works hand in hand with insurance executives to provide actionable insights using data, analytics, and consultative direction for their claims organizations. Ryan earned a Master’s degree from Northern Arizona University and a Bachelor’s degree from the University of San Diego. In 2015, he was selected as was one of the top 40 Business and Community Leaders under the age of 40 by Business Examiner Magazine.
Abstract:
We all understand that data has rapidly become the new raw material of modern business. Analytics have now permeated into all levels of the organizational hierarchy creating exciting new opportunities but also some interesting challenges. Not all business units are equipped with data scientists and analytics experts to help team members navigate these uncharted waters that are flooded with information. The challenge we face is making data accessible to a wide range of stakeholders that have little to no experience in the field of data science so that our organizations can achieve the greatest value and impact from both our data and human resources. This talk will focus on strategies to bring data into the greater culture of a business and integrate analytics into all levels of personnel regardless of technical experience.
Joshua New
Oak Ridge National Laboratory, USA
Title: Big Data Mining for Applied Energy Savings in Buildings

Biography:
Dr. Joshua New completed his Ph.D. in Computer Science at the University of Tennessee, USA, in 2009. He is an R&D staff member of Oak Ridge National Laboratory and currently serves at ORNL’s Building Technology Research Integration Center (BTRIC) as subprogram manager for software tools and models. He has over 100 peer-reviewed publications and has led more than 45 competitively-awarded projects in the past 5 years involving websites, web services, databases, simulation development, visual analytics, supercomputing using the world’s #1 fastest supercomputer and artificial intelligence for big data mining. He is an active member of IEEE and ASHRAE.
Abstract:
Residential and commercial buildings in China, India, the United States (US), United Kingdom (UK), and Italy consume 39-45% of each nation's primary energy (approximately 73% of electricity). Building energy models can be used to automatically optimize the return-on-investment for retrofits to improve a building’s energy efficiency. However, with an average of 3,000 building descriptors necessary to accurately simulate a single building, there is a market need to reduce the transaction cost for creating a simulatable model for every building in a city and calibrate the models to utility data prior to capital expenditures.
Oak Ridge National Laboratory has utilized two of the world’s fastest supercomputers, assembled unique datasets, and developed innovative algorithms for big data mining to produce the Automatic detection and creation of Building Energy Models (AutoBEM) technology for urban-scale energy modeling. The project developed the world’s most accurate method for determining building footprints from satellite imagery and the world’s fastest building energy model generator. The team has also leveraged a total of eight supercomputers to analyze the best methods, metrics, and algorithms for calibrating building models to measured data within 4% of hourly electricity use; well beyond current industry standards necessary for private-sector financing. The project developed the world’s fastest buildings simulator, over 8 million simulations totaling over 200TB, and mined this data with over 130,000 parallel artificial intelligence algorithmic instances to develop the world’s best calibration algorithm in terms of accuracy, runtime, and robustness.
Petra Perner
Institute of Computer Vision and applied Computer Sciences, IBaI, Leipzig
Title: New Ways to present the Results of a Data Mining Process to an Expert
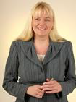
Biography:
Petra Perner (IAPR Fellow) is the director of the Institute of Computer Vision and Applied Computer Sciences IBaI. She received her Diploma degree in electrical engineering and her PhD degree in computer science for the work on “Data Reduction Methods for Industrial Robots with Direct Teach-in-Programing”. Her habilitation thesis was about “A Methodology for the Development of Knowledge-Based Image-Interpretation Systems". She has been the principal investigator of various national and international research projects. She received several research awards for her research work and has been awarded with 3 business awards for her work on bringing intelligent image interpretation methods and data mining methods into business. Her research interest is image analysis and interpretation, machine learning, data mining, big data, machine learning, image mining and case-based reasoning.
Abstract:
Data Mining methods can easily work on applications with many features and come up with decision rules, relations or patterns for the application. The results should be reported in such a way that an expert can overlook them. Often it is forgotten that the quality of the presentation depends on the kind of attributes you are using and for e.g. the decision tree induction method. Therefore, attribute construction or summarization in a way that many attributes represent a feature is necessary. We show on different applications how this can be done and what quality of representation can be achieved. We also show what kind of representation binary and n-ary decision tree induction methods can bring out.
Iqra Jahangir
Abasyn University Islamabad Campus, Pakistan
Title: Comparison of Random Tree and J48 for the Prediction of Dengue Fever through Data Mining
Biography:
Iqra joined the Abasyn University Islamabad Campus in 2014 for the Bachelor’s degree in Software Engineering, Iqra has been enrolled in the department of Computing and Technology. For the completion of her degree, Iqra is working at a Research project as her Final year project related to the Prediction of Dengue fever by using Data Mining techniques.
Abstract:
Data mining is a procedure, which is used to renovate useless data into useful information. Multiple techniques are a part of data mining which can be used in various fields. Most important purpose for me is Healthcare. Dengue, is a threatening ailment and it is triggered by the bite of a female mosquito. Pakistan has been a prey of this ailment from a couple of years. Life of many people is scarce from it. Lives of the peoples can be protected if the dengue is predicted in early stages. This research will present a dengue prediction methodology by comparing data mining technique such as J48 and Random tree. First, the data was gathered then it was used in Weka data mining tool by applying J48 and Random Tree. The results derived from these classifiers were supplementary used for the prediction of dengue. Generated results were able to identify that Random tree shows higher accuracy as compared to J48.